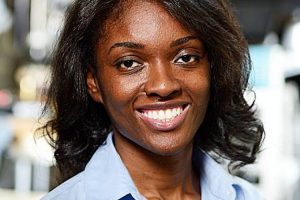
The following was originally published in The Hub.
Photoacoustic imaging is an imaging modality related to ultrasound imaging. A light source is inserted into a hollow-core needle and used to illuminate the patient’s tissues. The tissue expands, resulting in pressure waves, which are received by an ultrasound probe and then converted into human-interpretable images using a process called beamforming.
Unfortunately, images produced through beamforming can often be unclear and riddled with “artifacts”: phantom images that make it difficult for humans to interpret what is actually there.
Mardava Gubbi, a doctoral candidate in the Department of Electrical and Computer Engineering, is working to improve the traditional photoacoustic imaging process by bypassing beamforming altogether.
His approach involves integrating a photoacoustic imaging system, a deep learning-based tracking system, and a robotic arm to track needle tips in different imaging environments during surgery.
The technology is available for licensing through Johns Hopkins Technology Ventures.
“This research is notable for two reasons,” Gubbi said. “First, the idea of using photoacoustic images as inputs to a robotic visual servoing system—which refers to using information extracted from images to control the motion of robotic systems—is new and has multiple advantages over the traditional method of ultrasound imaging. Second, using the outputs of a deep learning-based system as inputs to our robotic visual servoing process allows for improved tracking of needle tips compared to creating a human-interpretable image and extracting the needle tip position from that image.”
Deep learning refers to a family of machine learning algorithms capable of extracting information from raw inputs such as images. With Gubbi’s work, the visual component consists of a photoacoustic imaging system providing raw sensor data to the deep learning system, which then extracts the position of the needle tip in the raw sensor data frame. The coordinates of the needle tip position are then provided to the robotic control system, which gives commands to move the robot to track the needle tip.
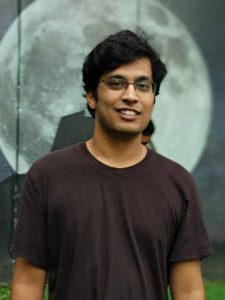
Mardava Gubbi
Gubbi says that this research has the potential to improve surgical procedures such as biopsies and catheter insertions by automating the task of tracking needle and catheter tips and providing doctors with information regarding the surrounding tissue. He believes it should also reduce the risk of surgical complications, such as bleeding, accidental injury to nearby critical organs, and sepsis from these procedures.
A member of Assistant Professor Muyinatu Bell‘s Photoacoustic & Ultrasonic Systems Engineering (PULSE) Lab, Gubbi says his approach will be of particular benefit to obese patients, as photoacoustic imaging is better suited to track needle tips in those patients compared to traditional ultrasound imaging, which often results in “noisier” images in patients with larger body sizes.
“Our process halves the distance traveled by the wave to the ultrasound probe, resulting in stronger received signals and reduced noise in photoacoustic images,” he said. Gubbi presented this work in November at the 2021 Maryland STEM Festival, which focused on applications of technology and robotics in health care.
“This project combines advances in deep learning, robotics, and photoacoustic imaging to solve the general problem of tracking needle tips, catheter tips, and other surgical tool tips in tissue,” Gubbi said. “I started working on this project both due to its multidisciplinary approach as well as the wide range of applications, and I’ve thoroughly enjoyed pursuing it.”
Other technologies from Bell’s PULSE Lab are available for licensing through JHTV.