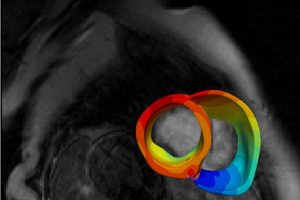
The following was originally published by Johns Hopkins University’s Office of Communications.
Johns Hopkins University scientists have developed a new tool for predicting which patients suffering from a complex inflammatory heart disease are at risk of sudden cardiac arrest. Published in Science Advances, their method is the first to combine models of patients’ hearts built from multiple images with the power of machine learning.
“This robust new personalized technology outperformed clinical metrics in forecasting future arrhythmia and could transform the management of cardiac sarcoidosis patients,” said senior author Natalia Trayanova, a Johns Hopkins professor of biomedical engineering and co-director of the Alliance for Cardiovascular Diagnostic and Treatment Innovation (ADVANCE).
Doctors don’t currently have precise methods for assessing which patients with carciac sarcoidosis, a condition causing inflammation and scarring that can trigger irregular heartbeats, are likely to have a fatal arrthmia, meaning that some patients don’t survive, while others undergo uncecessary, invasive interventions. A recent meta-analysis cited in the study found that roughly only one third of CS patients receive adequate treatment.
“There is an urgent clinical need for better predictive tools,” said Trayanova, who is also a professor at the Johns Hopkins School of Medicine. “Some CS patients perish, often in the prime of their life, while others have a defibrillator implanted unnecessarily and often deal with the complications, including infections, device malfunction, and inappropriate shocks, without receiving any real benefit.”
In their study, the researchers created digital three-dimensional models of the hearts of 45 CS patients treated at the Johns Hopkins Hospital. To do this, they took the novel approach of combining data from two different kinds of heart scans: contrast-enhanced cardiac MRIs, which detect fibrosis, or scarring, and PET scans, which detect inflammation.
“These personalized heart models are the first of their kind to be created with data from multiple imaging modalities,” said Trayanova, whose lab has pioneered techniques for mechanistic modeling of the heart. “The combined effects of fibrosis and inflammation have never previously been represented in heart models.”
The team used computer simulations to apply a series of electrical signals at various locations throughout each of the models and gathered millions of data points measuring each heart’s reaction.
“We gathered extremely high-dimensional data with the aim of understanding how various features of the scarring and inflammation affected the heartbeat,” said Trayanova.
The team then combined data from the mechanistic simulations, along with additional patient and imaging data, to develop and train an algorithm to predict the likelihood of arrhythmia leading to cardiac arrest.
“In a complex disease such as CS, with both scarring and inflammation, learning on mechanistic simulation results allowed us to relate them to real-world outcomes,” said Julie Shade, the lead author of the study and a PhD candidate in biomedical engineering in Trayanova’s lab.
The tool significantly outperformed standard clinical metrics for predicting cardiac arrest in CS patients.
To refine the algorithm, the team also conducted an intensive process of cross-validation, which assesses whether the same precision is achieved when different subsets of the data are removed, suggesting how the tool may perform on future patients. All in all, the team conducted 560 iterations of cross-validation.
“We were able to estimate the tool’s accuracy for new patients with 95 percent confidence, meaning we were relatively certain the algorithm wouldn’t be biased by the data it was trained with and that it would therefore be accurate when applied to new patients,” said Shade.
Lastly, the team compared their simulations against scans of lesions in the hearts of the patients who had subsequently undergone a procedure to reset their heartbeats, finding that their predictions were consistent with actual outcomes.
Large clinical studies will be needed, but the team hopes their tool could transform the management of patients with CS, lowering the number of unnecessary defibrillator device implantations while ensuring that patients at risk of sudden cardiac death are protected.
According to Trayanova, the synergistic use of personalized models and machine learning, which has never previously been used to address a problem in cardiovascular healthcare, could also help solve one of the biggest challenges to implementing artificial intelligence in healthcare: a lack of data.
“To achieve precision, AI-powered disease prediction technologies typically require vast quantities of data from different pools of patients,” said Trayanova. But in this case the complexity of the data from the heart models streamlined the learning process. “Because the heart models embody well-established, deterministic principles that do not need to be learned by the algorithm, the risk predictor can achieve excellent performance on small patient cohorts,” she said.
Authors included: Adityo Prakosa, Dan M. Popescu, and Rebecca Yu of Johns Hopkins University and David R. Okada and Jonathan Chrispin of Johns Hopkins University School of Medicine.
This work was supported by NIH grants R01HL142496 and R01HL126802 AQ18, Lowenstein Foundation, Robert E. Meyerhoff Professorship, Johns Hopkins University Undergraduate Research Fellowship, and NSF Graduate Research Fellowship DGE-1746891.