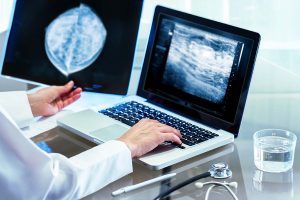
The following was originally published on the Johns Hopkins Applied Physics Lab.
Throughout his nearly three decades at the Johns Hopkins Applied Physics Laboratory (APL) in Laurel, Maryland, Dave Porter has contributed to work ranging from ballistic missile accuracy to intelligence surveillance and even groundwater remediation.
But in December 2011, his wife, Pat, received a dreaded diagnosis: breast cancer — and a very aggressive form of it.
“It was so small and so early, it very easily could’ve been missed,” said Porter, a modeling and estimation engineer at APL.
That’s when his personal and work worlds collided. Porter and a dedicated team of co-inventors had previously developed and patented a technology called upstream data fusion (UDF). UDF leverages artificial intelligence (AI) to combine multiple sources of data — ultimately conveying better information than any one source could provide.
“The first level of UDF technology is for finding objects,” Porter explained. “On a battlefield, it might be used to find surface-to-air missiles, for example. But in a medical setting, it applies to the kinds of diseases that are characterized by anomalous tissue or nodes. We realized this technology could be used to detect lung diseases or cancers.”
After his wife’s diagnosis, Porter led a team to adapt UDF technology for a new fight.
“My wife kept saying to me, ‘You’re always talking about finding bad guys on a battlefield, why don’t you find a bad lesion in a woman’s breast?’” Porter recalled emphatically.
Improving Breast Cancer Detection
Although preventative screening has reduced breast cancer mortality rates, the systems for detection are not perfect. Present-day mammography screening is expensive and requires significant medical infrastructure. Ultrasounds are often recommended for women with dense breast tissue but sometimes produce false positives and prompt unnecessary biopsies. Magnetic resonance imaging (MRI) is also used to detect breast cancer, but it is of limited availability, expensive and ultimately considered the “Cadillac” modality, according to Porter.
“All of these systems and the staff that use them are stovepiped,” Porter said. “They are separate disciplines and have limited interaction with one another. Unfortunately, when that happens, information gets lost.”
Further, each breast cancer screening method produces multiple images, views and angles of a patient’s breasts. It begs the question: How can radiologists automate their processes to improve accuracy and efficiency?
“When a radiologist sits at their workstation and sees multiple views and images from a breast screening, they must do mental gymnastics to figure out where a lesion should be in another image of the same breast,” Porter said. “Our fusion technology can help by looking for anomalous tissue that is similar in location but also similar in characteristics.”
A Key Breakthrough
Realizing there was a gap to fill on the front lines of breast cancer detection, Porter teamed up with APL senior image processing engineer William Walton, mathematician Michael Williams, radiologist Dr. Lisa Mullen of Johns Hopkins Medicine, radiologist Dr. Susan Harvey, formerly of Johns Hopkins Medicine, and independent consultant Keith Peyton to study how data fusion and machine learning could be applied to optimize breast cancer detection efforts.
Walton has spent much of the past 27 years writing computer algorithms to find objects in satellite images. He was able to provide a missing piece to UDF: his patented convolutional neural network image registration, which was also the focus of his recent Ph.D. dissertation at the University of Maryland, Baltimore County.
Image registration provides the ability to map multiple views of the same object so that they align with one another. When image registration is combined with UDF technology, the team can register the location of a potential breast lesion in a corresponding view more quickly and with more accuracy. Porter describes the collision of these two technologies as “magic.”
“In both the military setting and the doctor’s office, experts spend their time looking back and forth at different views of the same thing,” Walton said. “Our research shows that if we combine multiple views and pinpoint potential lesions, we can improve performance of detection.”
The team’s tireless efforts paid off this year when APL’s Tech Transfer signed a commercial option with Curing Women’s Cancer, an Arizona-based foundation, to perform a commercial feasibility study on incorporating the APL-patented data fusion and image registration technologies into an AI-assisted, low-cost, portable ultrasound screening solution for breast cancer detection. The intent is to lower costs, improve outcomes and broaden access to breast cancer screening in underserved parts of the United States and around the world.
“An experienced radiologist once told me she often interprets images for 90-100 women a day,” Walton said. “Clinicians know that what they convey to a patient matters and they want to get it right. That’s what our technology is doing — trying to help them get the diagnosis right, and more quickly.”
The APL researchers say they’re hopeful to continue this research and ultimately submit their technologies to the U.S. Food and Drug Administration for clearance.
Making a Difference
For Porter and Walton, it’s been fulfilling and invigorating to apply technology typically used with satellites and in military settings to better equip doctors on the front lines of breast cancer detection.
According to Porter, his wife credits the radiologist from her 2011 breast screening with saving her life. After extensive treatment, she’s remained cancer-free for more than a decade.
“This is the best of all the things I’ve been involved with in my career — and I’ve been involved in some incredible stuff,” Porter said.
And, the work continues with the hope to save the lives of future patients.
“We want to make a difference,” Porter said.