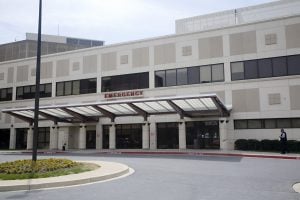
When a patient arrives in any emergency department, one of the first steps in their care process is triage, an opportunity for a care team member to identify critically ill patients and assign priority treatment levels.
“With increases in annual visits to U.S. emergency departments, declines in capacity have led to unprecedented levels of crowding and consequential delays in care,” says Scott Levin, Ph.D., associate professor of emergency medicine at the Johns Hopkins University School of Medicine. “So what emergency departments have to do is very quickly assess whether a patient is in need of real critical, time-sensitive treatment versus a patient who is safe to wait.”
Across the country, nurses and physicians typically use the emergency severity index (ESI) during triage to assign a score from Level 1 for patients who are the most critically sick, to Level 5 for patients who are the least sick. A patient’s ESI level determines in which area of the emergency department that patient will be seen, places the patient in a queue and influences provider decision-making throughout the patient’s care process. “This algorithm is completely subjective,” Levin says. “Nurses and physicians make a quick assessment on whether the patient can wait solely based on their clinical judgment.” In most cases, researchers say patients are assigned to a Level 3 and not entirely differentiated. “We thought that Level 3 patient group included a large mix of patients who are pretty sick and others who weren’t, and our goal was to determine whether these patients could be sorted out,” Levin says.
To help differentiate patient triage levels, Levin and a team in the Department of Emergency Medicine developed an electronic triage tool. In a recently published paper in the Annals of Emergency Medicine, the e-triage tool showed equal or improved identification of patient outcomes compared to ESI based on a multi-site retrospective study of nearly 173,000 emergency department visits. The study showed significant differences in patient priority levels using e-triage and ESI. For example, out of the more than 65 percent of visits triaged to ESI Level 3, e-triage identified about 10 percent, or more than 14,000, ESI Level 3 patients who may have benefitted from being up-triaged to a more critical priority level, such as Level 1 or 2. These patients were at least five times more likely to experience a critical outcome, such as death, admission to the ICU or emergency surgery, and two times more likely to be admitted to the hospital. The e-triage tool was also able to increase the number of patients down-triaged to a lower priority level, such as Level 4 or 5, to help minimize low-acuity patients from waiting and overusing scarce resources.
The e-triage tool uses an algorithm to predict patient outcomes based on a systems engineering approach and advanced machine learning methods to identify relationships between predictive data and patient outcomes. “When a patient comes in, and we collect the patient’s information, the e-triage tool is comparing that patient to hundreds of other like patients to make a prediction on the patient’s outcome,” Levin says.
These methods are common in other industries, such as defense, transportation and finance, but rarely, if ever, are implemented in health care. “Machine-based learning takes full advantage of electronic health records and allows a precision of outcomes not previously realizable,” says Gabor Kelen, M.D., director of the Department of Emergency Medicine and professor of emergency medicine at the Johns Hopkins University School of Medicine. “It is the wave of future health care, although some providers may be hesitant. Decision aids that take advantage of machine-learning are also highly customizable to meet the needs of an emergency department’s patient population and local health care delivery systems.”
The e-triage tool is also designed to be a decision support tool to help clinicians make better care decisions about their patients. “The theory behind this tool, and all clinical decision support tools, is that the tool paired with the clinician can make better predictions or better prognostics tasks like this than the tool alone or the clinician alone,” Levin says.
Better differentiating patients’ priority levels, can, in turn, help patients get the appropriate care they need. “The ultimate objective is patients should be waiting less in the emergency department,” Levin says. “For patients at risk of having a critical care need, this technology is designed to detect them better and make sure they are seen quicker. For patients who are less sick, e-triage should detect those patients and put them on an expedited track, so they don’t need to wait as long.”
E-triage is currently being used at The Johns Hopkins Hospital and Howard County General Hospital, both member hospitals of Johns Hopkins Medicine. The tool is continuing to be prospectively evaluated with preliminary results that suggest improvement in detection of patients with critical outcomes and other measures relating to emergency department crowding, according to researchers.
Other researchers involved in this study include Matthew Toerper, B.S.; Eric Hamrock, M.B.A.; Jeremiah S. Hinson, M.D., Ph.D.; Sean Barnes, Ph.D.; Heather Gardner, R.N.; Andrea Dugas, M.D., Ph.D.; Bob Linton, M.D.; and Tom Kirsch, M.D., M.P.H.
Funding for this study was provided by the Agency for Healthcare Research and Quality (AHRQ) (grant R21HS023641) and the National Science Foundation (NSF) (grant SBIR 1621899).
Under a license agreement between StoCastic, LLC and The Johns Hopkins University, Levin, Dugas, Kelen and Toerper are entitled to royalty distributions on technology described in this publication. Levin is also a founder and holds equity in StoCastic, LLC. Toerper is a paid consultant to StoCastic, LLC. Barnes is an employee of StoCastic, LLC. These arrangements have been reviewed and approved by The Johns Hopkins University in accordance with its conflict of interest policies.